In a research driving simulator the effects of time-on-task were measured on variables that measure drowsiness, driving performance and steering behaviour. It was found that the fraction of time during which the eyes are closed is a good measure of drowsiness that is sensitive to the effects of time-on-task. Of all single variables that measure driver performance and impairment, the percentage of time during which any part of the vehicle exceeded one of the lane boundaries was the most strongly affected by time-on-task. Also, with progressing drowsiness, the amplitude of steering corrections increased towards larger values. This was caused both by larger error corrections in response to larger errors and by an increase in coarseness of the steering response. Large steering corrections proved to be the single best indicator of progressing impairment by drowsiness and fatigue. 1. INTRODUCTION
Falling asleep at the wheel and drowsiness are considered important factors in accident causation. Estimates of the involvement of these factors in accidents are higher when the statistics are based on in-depth accident studies (10-25%) compared to statistics based on general police databases (1-4%) (Horne & Reyner, 1995). This indicates that, although the scope of the problem is not clear, drowsiness and fatigue are significant risk factors. Because of this a large number of studies on drowsiness, fatigue and sleepiness have been reported in the literature. These studies differ widely in the variables used to measure drowsiness and impaired driving.
Drowsiness is a psychophysiological state that is assumed to result in an inability of the driver to drive safely. Drowsiness is often measured by eyelid closures. The percentage of time the eyes of the driver are 80 to 100% closed (PERCLOS) has been used as a variable for measuring drowsiness. Dingus, Hardee and Wierwille (1987) found that PERCLOS correlated better with driver impairment than other measures of eyelid closures that were examined. The use of eyelid closures as an indicator of drowsiness stems from the general observation that as people get drowsy they close their eyes more frequently and during longer periods, until they finally fall asleep. However, in an experiment by Wierwille, Lewin and Fairbanks (1996) it was found that PERCLOS did not predict drifting off the road very well. Since measures of eyelid closure were not sensitive enough, they advised to monitor lane position as well in order to detect driver impairment.
A number of other studies have focussed on driver behaviour instead of driver state. According to Bishop, Madnick, Walter and Sussman (1985), steering activity becomes more coarse when driving for long periods of time: the number of large steering movements increases while the number of smaller steering amplitudes decreases. Seko, Kataoka and Senoo (1985) also found that with reduced alertness caused by drowsiness, the number of steering corrections with large amplitudes increases. This suggests that with progressing drowsiness the number of lapses of attention increases resulting in longer periods during which there are no steering corrections. This would result in drifting to the edge of the lane which is corrected by a larger steering amplitude. This view is consistent with the theory of ‘blocking’ as proposed by Bertelson and Joffe (1963). They found that with progressing fatigue the occurrence of ‘blocks’ of large reaction times increases. After a ‘block’, performance returns to normal for a while. A blocking may express itself as a failure to commit a correcting steering action in time which results in smaller safety margins to the lane boundary, crossing the lane boundary or in moving off the road. In that case the occurrence of an error (i.e. a smaller safety margin or a lane boundary exceedance) is not the only indication of impaired performance because of drowsiness. Also the increase in the number of large steering amplitudes is an indication of error correction in response to a larger error. This error correction response then evidences progressing impairment. Error correction may then be defined as turning the steering wheel in the opposite direction, with a large peak-to-peak amplitude, when the driver notices that the lane boundary is about to be crossed or has been crossed. Error corrections prevent accidents to occur and they may partly prevent effects of drowsiness on measures such as the standard deviation of the lateral position (SDLP) or exceedance of the lane boundaries. From this perspective it may be that effects on steering amplitude-related measures as indicators of error correction show up earlier than effects on lane position related variables. However, with progressing drowsiness, error corrections may come too late resulting in increased swerving or running off the road.
This reasoning assumes that safety margins to the lane boundary are perceived by the driver and acted on by a correcting steering action. This principle has been demonstrated by Godthelp (1988) in a study where drivers were instructed to generate correcting steering actions when vehicle heading could still be corrected comfortably to prevent a crossing of the lane boundary. Safety margins were defined by the concept of Time-to-Line Crossing (TLC). This represents the time available until any part of the vehicle reaches one of the lane boundaries. This coupling of perception and action has also been demonstrated for driving in curves by Van Winsum and Godthelp (1996) and for the way drivers change lanes by Van Winsum (in press).
Another group of studies has focussed on the effects of fatigue on driver performance instead of psychophysiological measures and measures of driver control behaviour. The standard deviation of the lateral position (SDLP) and exceedance of the lane boundaries are generally referred to as indicators of the quality of driving behaviour and, thus, driver performance. It has been found that SDLP increases with time on task (Riemersma, Sanders, Wildervanck & Gaillard, 1977; De Waard & Brookhuis, 1991). SDLP measures swerving and control over lateral position. Another measure of driver performance is the proportion of time that any part of the vehicle exceeds the lane boundary. This has been referred to as ‘LANEX’ by Wierwille, Lewin and Fairbanks (1996). LANEX is a strong indicator of driver impairment. The minima of TLC are used in the present study as an additional measure of driver performance. These minima represent the safety margins to the lane boundaries that are maintained by the driver. Smaller TLC minima indicate poorer lateral control and suggest poorer driver performance and progressing impairment.
In summary, three types of variables are used in the present study. Variables that measure driver performance are LANEX, SDLP and TLC minima. Other variables measure drowsiness. These are related to eyelid closures, especially PERCLOS (i.e. the percentage of time that both eyes are closed). The third type of variable relates to driver behaviour or more specifically to steering behaviour of the driver.
The experiment was performed in the driving simulator of the TNO Human Factors Research Institute.
2. METHOD
Eighteen paid subjects participated in the experiment. Age ranged between 24 and 70 years. All subjects had held their drivers licence for more than 5 years and the annual kilometrage exceeded 5000 km. The experiment was performed in the driving simulator of the TNO Human Factors Research Institute, described in detail in Hogema and Hoekstra (1998), and Hoekstra, Van der Horst and Kaptein (1997).
For the detection of eyelid closures, EOG was measured for both eyes with electrodes attached just above and below each eye in line with the pupil. By this procedure the signal is affected only by artefacts caused by vertical eye movements and not by eye movements in the horizontal plane. The electrodes were connected to a physiological amplifier with a timeconstant of 10 s. The amplifier gave an output between –5 and +5 Volts. This was fed directly into the A/D converter of the simulator computer, where it was sampled and stored together with the driver behaviour data. This ensured a fixed synchronization in time between all signals.
All subjects were informed about the purpose of the experiment and that it took three hours of continuous driving. Subjects were free to stop driving at any moment without negative consequences. They were requested to stop if they were feeling uncomfortable or if they were too tired to continue to drive safely. After the instructions, the electrodes for EOG measurement were attached and the signal was tested with an oscilloscope. During the drive, speed was controlled by a cruise control that was set at a constant speed of 80 km/h. This was expected to facilitate the occurrence of drowsiness because of the relatively low speed in a visually boring environment. All subjects drove continuously for three hours during the daytime on a monotonous road under simulated evening lighting conditions. No other traffic was encountered. The road was a standard two-lane road with a lane width of 3.1 m, broken centerline and continuous edgelines. It consisted of straight and curved segments with a continuous horizontal radius of 2000 m that turned either to the left or to the right over an angle of 45º. Subjects were instructed to drive in the right lane without exceeding the lane boundaries. A mild side wind with varying force was simulated in order to necessitate a minimum amount of steering effort.
Coordinate positions were stored as well as steering wheel angle, lateral position and EOG recordings of both eyes with a frequency of 10 Hz. Time-to-Line Crossing (TLC) was computed off-line according to the method described in Van Winsum, Brookhuis and De Waard (in press). Time-on-task (TOT), i.e. the effect of progressing time that is assumed to result in fatigue and drowsiness, was treated as a within-subjects factor as follows. The first 20 minutes of each run was not analyzed since this period was used to familiarize the subjects with driving in the simulator. After this, the remaining time was divided into 5 sequential blocks of equal duration. Usually these blocks each covered a period of 32 minutes. This means that there are 5 TOT blocks. Effects of fatigue and drowsiness on task performance are expressed as an effect of TOT. To evaluate time-on-task effects the dependent variables were averaged over each block and divided by the average value for the first block where appropriate. In this way all variables have the same units and can be compared directly in terms of the sensitivity to TOT effects. If it is assumed that drowsiness increases with increasing time on task, then the variables with the strongest statistical effect of TOT are most useful as indicators of drowsiness. This procedure of dividing the average by the data of the first time block can only be used if the data on the first block can never be zero. Therefore, not all variables are suitable for treatment by this procedure.
EOG data were filtered off-line and the filtered signal was subtracted from the raw EOG signal to allow peak detection analysis by a computer program that detected eyeblinks and eyelid closures. These were transformed into the following indicators of drowsiness:
- PERCLOS, i.e. the fraction of time during which both eyes are closed
- BLINK, i.e. the blinkfrequency
For each TOT block, the average value was divided by the value of the first block.
The following indicators of driver performance were computed:
- LANEX, i.e. the fraction of time during which any of the wheels exceeds the right lane boundary.
- SDLP, i.e. the standard deviation of lateral position, with respect to the first block.
TLC minima to the left and right lane boundaries were determined and only minima of less than 20 s were analyzed. These minima were used to compute:
- TLC1.0, i.e. the percentage of TLC minima smaller than 1.0 s.
The following indicators of steering behaviour were computed:
- SDST, i.e. the standard deviation of steering wheel angle, with respect to the first block,
- P3-6, i.e. the power of fast steering movements (in the domain of 0.3-0.6 Hz) as a fraction of all steering activity < 0.6 Hz, with respect to the first block.
- STAMP, i.e. the average of peak-to-peak steering amplitudes, with respect to the first block
- STDIS, i.e. the fraction of larger peak-to-peak steering amplitudes. This was computed as follows: for the first block the distribution of all peak-to-peak steering amplitudes was computed and the 80th percentile value (i.e. that value for which 80% of all values are smaller and 20% of all values are larger) was determined. Then, for all subsequent time blocks the percentage of values that was larger than the initial 80th percentile value was computed and divided by 20 (i.e. the percentage larger than the value in the first block). Figure 1 gives a graphical illustration of this principle. There are two bell-shaped distributions of peak-to-peak steering amplitudes. The left distribution refers to the first block, while the right distribution represents block i. The idea then is that as drowsiness progresses, the distribution of steering amplitudes shifts towards larger values (to the right). The vertical line represents the value of steering amplitudes, during the first block, that separates the 20% largest values from the 80% lowest values. The sum of the striped and black area represents the percentage of values that is larger than P80 of the first block. STDIS then is the sum of the black and striped area divided by the black area.
The following types of analyses were conducted:
1) The effect of time on task was tested with analysis of variance using a within-subjects repeated measurement design. The aim of these analyses was to evaluate the relative sensitivity of the dependent variables to effects of drowsiness.
2) For each block the magnitude of the TLC minima was related to the correcting steering wheel action in the opposite direction. This was realized as follows. TLC minima to the right lane boundary result in a path-correcting turning of the steering wheel to the left, while TLC minima to the left lane boundary result in a path-correcting turning of the steering wheel to the right. All TLC minima to either the left or the right were detected together with the accompanying correcting peak-to-peak steering amplitude to the opposite direction. Then the TLC minima were categorized into groups according to the magnitude of the TLC minima. For testing the relation between TLC minima and steering corrections, the following groups were distinguished:
1 =TLC minima >0.0 and <=1.5 s; 2 =TLC minima >1.5 and <=3.0 s
3 =TLC minima >3.0 and <=4.5 s; 4 =TLC minima >4.5 and <=6.0 s
5 =TLC minima >6.0 and <=7.5 s; 6 =TLC minima >7.5 and <=9.0 s
7 =TLC minima >9.0 and <=10.5 s; 8 =TLC minima >10.5 and <=12.0 s
9 =TLC minima >12.0 and <=13.5 s; 10 =TLC minima >13.5 and <=15.0 s
A smaller TLC minimum can be considered as a larger error that is compensated by a larger steering correction (larger peak-to-peak steering amplitude in the opposite direction). Analyses of variance were applied to test whether the sensitivity of the steering response to TLC information changes as a function of time on task.
Figure 1. Distributions of peak-to-peak steering amplitudes. STDIS is computed as the sum of the black and striped area divided by the black area.
3. RESULTS
Table 1 gives an overview of the effects of time on task on the different dependent variables. It can be seen that all variables related to driver performance, drowsiness and steering behaviour are strongly affected by time of driving: performance deteriorates with increased driving time, and drivers become more drowsy while their steering becomes more coarse. The steering amplitude related variables, i.e. STDIS and STAMP, have the largest effect of time on task. This means that the effect of time-on-task on these variables is the most reliable, as indicated by the F-statistic. When comparing STDIS and STAMP, the effect size of time-on-task is the largest for STDIS. This can also be seen in figure 2. These results indicate that, of all variables examined in the present experiment, the fraction of large peak-to-peak steering amplitudes (STDIS) is the best indicator of drowsiness-related driving impairment.
Table 1. Effects of time on task on the dependent variables (F-statistics), together with average values
| Time on task block |
Type | Dependent variable | Effect of time on task df=68,4 | 1 | 2 | 3 | 4 | 5 |
Driver performance | | | | | | | |
| LANEX | 8.81 ** | 0.02 | 0.05 | 0.06 | 0.08 | 0.08 |
| TLC1.0 | 7.34 ** | 4.48 | 6.33 | 6.14 | 6.78 | 7.14 |
| SDLP relative | 8.30 ** | 1.00 | 1.17 | 1.29 | 1.31 | 1.38 |
Drowsiness | | | | | | | |
| PERCLOS | 5.90 ** | 1.00 | 1.24 | 1.63 | 1.91 | 2.14 |
| BLINK | 9.51 ** | 1.00 | 1.20 | 1.43 | 1.48 | 1.58 |
Steering behaviour | | | | | | | |
| STDIS | 14.60 ** | 1.0 | 1.38 | 1.62 | 1.77 | 1.83 |
| STAMP | 15.25 ** | 1.00 | 1.09 | 1.18 | 1.22 | 1.25 |
| SDST | 11.49 ** | 1.00 | 1.09 | 1.11 | 1.20 | 1.20 |
| P3_6 | 9.00 ** | 1.00 | 1.04 | 1.08 | 1.13 | 1.12 |
** p < .001
From the previous analyses it appeared that with progressing drowsiness driver errors increased which resulted in more frequent crossing of the lane boundaries and smaller TLC minima. This may explain the shifting to larger steering corrections with time-on-task, since larger errors may be corrected by larger corrections. In order to test this, the magnitude of the TLC minima was related to the correcting steering wheel action in the opposite direction for each block according to the procedure described in paragraph 2. Analyses of variance were applied to test whether the sensitivity of the steering response to TLC information changes as a function of time on task. In these analyses the time-on-task effects of block 1 vs block 5 were tested. The effect of time-on-task on the amplitude of the steering corrections was significant (F(17,1)=30.43, p<.001), while the effect of TLC minimum on the amplitude of the corrective steering actions was significant as well (F(153, 9)=135.89, p<.001). This is illustrated in figure 3.
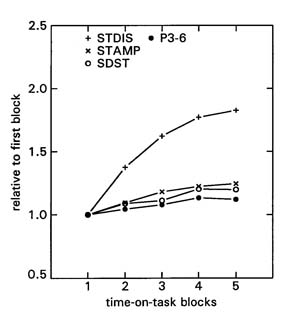
Figure 2. Variables related to steering behaviour as a function of time on task.
The results show that the magnitude of the correcting steering action is strongly related to the magnitude of the error, since smaller TLC minima are associated with larger correction peak-to-peak steering amplitudes in the opposite direction. However, in addition the effect of time-on-task of steering amplitude is highly significant, despite the fact that it has been controlled for TLC minima. This means that although the increase in larger steering corrections with time-on-task can partly be explained by the increase in errors (small TLC minima) with progressing time-on-task, there still is a substantial increase in the magnitude of peak-to-peak steering corrections which cannot be explained by larger errors. This suggests that as drowsiness increases, steering reactions to a movement of the car towards the lane boundaries become larger.
Figure 3. Peak-to-peak steering amplitude as a function of TLC minima for the first and the last time-on-task blocks.
4. CONCLUSIONS AND DISCUSSION
In an experiment performed in the TNO driving simulator the effects of drowsiness on several measures of driving performance, drowsiness and steering behaviour were studied. The method used was prolonged driving under monotonous environmental conditions. The results reveal significant effects of time-on-task on variables that measure drowsiness. These variables were based on eyelid closures derived from EOG measurements. Both the fractions of time during which both eyes were closed (PERCLOS) and the frequency of eyeblinks (BLINK) were significantly affected by time-on-task. This suggests that the experimental setup resulted in the desired effect of inducing drowsiness in the subjects. In accordance with the literature, PERCLOS appears to be a valid indicator of drowsiness. This variable is easy to compute from the data of an eyelid monitor and may be a useful variable for driving impairment detection systems.
Driver performance was measured by means of variables that were derived from lateral position. Of all performance-related variables, the fraction of time during which any part of the vehicle exceeds one of the lane boundaries (LANEX) was the most sensitive to effects of time-on-task. Because of this, it is recommended to include this variable in systems that are aimed at driver impairment detection. Also, in accordance with the literature, the standard deviation of lateral position (SDLP) was significantly affected by time-on-task as were the TLC minima.
The results of the steering related variables show that the fraction of large peak-to-peak steering amplitudes that are larger than the 80th percentile value during the initial period of driving were the most sensitive to the effects of time-on-task. This indicates that the distribution of steering amplitudes shifts towards larger values with progressing time. This variable appears to be more sensitive than all other variables that were measured in this experiment, driver performance and drowsiness-related variables included. It is therefore recommended to include this variable in systems for detecting drowsiness-related driver impairment.
In a final analysis it was evaluated how the relation between the magnitude of the errors, measured by the TLC minima, and the magnitude of the correcting steering wheel movements to the opposite direction was affected by time-on-task. The shift to larger steering corrections with progressing time-on-task may partly be explained by the larger errors that are committed when drowsiness increases. It appeared that the amplitude of the correcting steering response is indeed strongly related to the momentary TLC minimum: a smaller TLC minimum is accompanied by a larger steering correction in the opposite direction. However, when corrected for the level of the TLC minima, steering corrections still increase significantly with time-on-task. This cannot be explained by larger errors with progressing fatigue. The results suggest that the larger steering corrections that occur with higher levels of drowsiness are both the result of larger errors and of increased coarseness of the steering responses. The mechanism responsible for this effect is unclear. A possible explanation may be that with progressing drowsiness drivers tend to look at a point on the road closer in front in an attempt to reduce visual input. This may then result in poorer lateral control. There is experimental evidence for the idea that lateral control performance deteriorates when the driver has less preview, as is the case when the driver looks at a point closer in front of the vehicle. For example, Tenkink (1988) studied the effects of sight distances of 27, 37 and 183 meters on lateral control performance. He found that a smaller sight distance resulted in a larger SDLP at a given speed. The hypothesis that drowsy drivers look at a point closer in front of the vehicle is consistent with the results of Kaluger and Smith (1970). They found, in a study of driver fatigue, that drivers looked closer in front of the vehicle after several hours of driving. Mourant and Rockwell (1972) have described this compensating strategy of fatigued drivers as a regression towards the visual scan behaviour of novice drivers, who also are characterized by looking closer in front of the car compared to experienced drivers. Alternatively, this change in visual scanning strategy may be an attempt to reduce the amount and complexity of visual input. However, this hypothesis needs to be tested in further research.
REFERENCES
- Bertelson, P. & Joffe, R. (1963). Blocking in prolonged serial responding. Ergonomics, 6, 109-116.
- Bishop, H., Madnick, B., Walter, R. & Sussman, E.D. (1985). Potential of driver attention monitoring system development (Report DOT HS 806 744). Springfield, VA: National Highway Traffic Safety Administration.
- Dingus, T.A., Hardee, L. & Wierwille, W.W. (1987). Development of models for on-board detection of driver impairment. Accident Analysis and Prevention, 19(4), 271-283.
- Godthelp, J. (1988). The limits of path error-neglecting in straight lane driving.Human Factors, 28, 211-221.
- Hoekstra, W. van der Horst, R. & Kaptein, N.A. (1997). Visualisation of road design for assessing human factors aspects in a driving simulator. Proceedings Driving Simulator Conference (DSC ’97), 8-9 September, Lyon, France.
- Hogema, J.H. & Hoekstra, W. (1998). Description of the TNO Driving Simulator (Report TM-98-D007). Soesterberg, The Netherlands: TNO Human Factor Research Institute.
- Horne, J.A. & Reyner, L.A. (1995). Sleep related vehicle accidents. British Medical Journal, 310, 565-567.
- Kaluger, N.A. & Smith, G.L. (1970). Driver eye-movement patterns under conditions of prolonged driving and sleep deprivation. Highway Research Record.
- Mourant, R.R & Rockwell, T.H. (1972). Strategies of visual search by novice and experienced drivers. Human Factors, 14, 325-335.
- Riemersma, J.B.J., Sanders, A.F., Wildervanck, C. & Gaillard, A.W. (1977). Performance decrement during prolonged night driving. In R.R. Mackie (Ed.), Viligance: Theory, operational performance and physiological correlates (pp. 41-58). New York: Plenum.
- Seko, Y., Kataoka, S. & Senoo, T. (1985). Analysis of driving behavior under a state of reduced alertness. JSAE Review, April, 66-72.
- Tenkink, E. (1988). Lane keeping and speed choice with restricted sight distances. In T. Rothengatter & R. de Bruin (Eds.). Road user behaviour: theory and research. Assen/Maastricht, The Netherlands: Van Gorkum
- Waard, D. de & Brookhuis. K.A. (1991). Assessing driver status: a demonstration experiment on the road. Accident Analysis and Prevention, 23(4), 297-307.
- Wierwille, W.W., Lewin, M.G. & Fairbanks, R.J. (1996). Research on vehicle-based driver status.performance monitoring, PART III (Report DOT HS 808 640). Springfield, VA: National Highway Traffic Safety Administration.
- Winsum, W. van & Godthelp, H. (1996). Speed choice and steering behaviour in curve driving, Human Factors, 38(3), 434-441.
- Winsum, W. van, Brookhuis, K.A. & Waard, D. de. (in press). A comparison of different ways to approximate time-to-line crossing (TLC) during car driving. Accident Analysis and Prevention.
- Winsum, W. van (in press). Lane change manoeuvres and safety margins. Transportation Research, Part F: Traffic Psychology and Behaviour.